AI and ML in Education
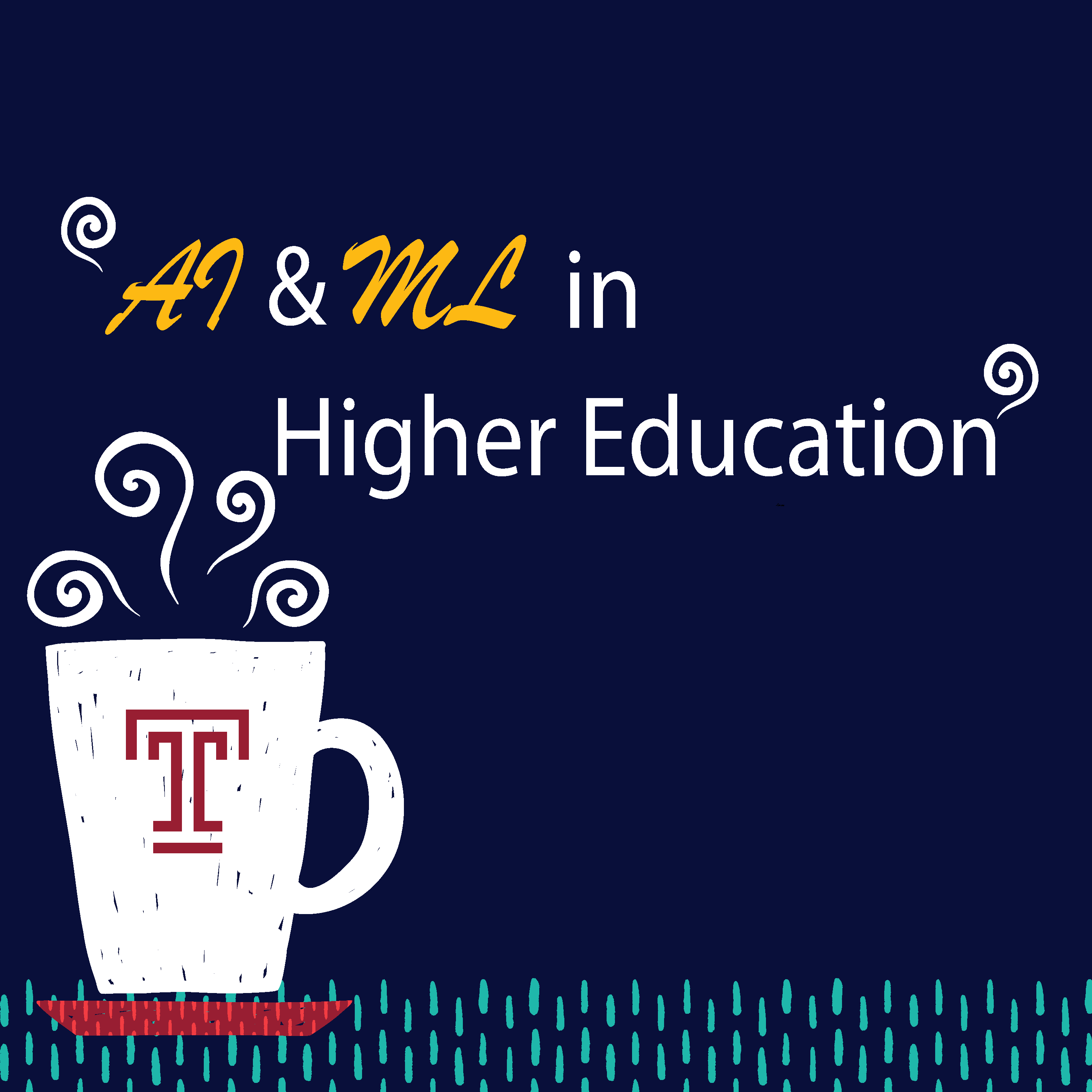
In this episode we revisit a topic from Season 1: Artificial Intelligence and Machine Learning. This time, we want to see how its being taught and the challenges it poses.
Show Transcript
Audio Transcript
Andrew ColettiHello and welcome to this episode of The T in Teaching. This episode is focused on a topic we covered in season one: Artificial Intelligence and machine learning. This time we wanted to discuss specifically how it is being taught and used in education. For this episode, I hosted three experts and applicators of AI and ML. Todd Schifeling, Konstantin Baumann and Jaeyeon Jeong.
Andrew ColettiDr. Todd Shiflett joined Temple University in 2017 as an assistant professor of strategic Management in the Fox School of Business. Prior to joining Temple, Dr. Schifeling earned his Ph.D. at the University of Michigan in sociology. Dr. Schifeling currently teaches a graduate level course called Getting Your Hands Dirty: The Craft of Data Management and Analysis, which helps graduate students use AI and ML to answer various questions in their field of research.
Andrew ColettiKonstantin Bauman joined Temple University back in 2018 after doing postdoctoral research at New York University. Bauman's research interests are primarily in areas of technical information systems, with focus on fields of quantitative modeling, data science, and specifically developing novel machine learning methods for predicting customer preferences. Jaeyeon Jeong is a Ph.D. student at the Fox School of Business Studying Information Systems.
Andrew ColettiHer primary research focuses on the economics of artificial intelligence and market design. Her and Konstantin Bauman recently published a study on the effectiveness of AI chat bots in educational assessments. Thank you for listening and please enjoy. Hello and welcome back to this episode of The T in Teaching. In this episode, we get to visit a topic we talked about in the first season, which is AI machine learning.
Andrew ColettiIn that episode, we talked a lot about what AI machine learning are, what the function and the future of that technology will be. But in this episode we want to revisit it, but instead talk more about how it's being taught and the challenges that it poses to the teachers and students alike. With me, I have three guests who are experts in AI machine learning.
Andrew ColettiIn fact, Konstantin, one of our guest hosts and runs a workshop every month for the Fox faculty on AI Machine Learning, which I was lucky enough to be able to attend. In it, one of the leading researchers Sudipta Basu, said, “We're opening up a new toolkit with AI/Machine Learning”, which I thought was a really interesting concept because inside of a tool kit are a lot of different things.
Andrew ColettiSo, let's start with this idea that A.I. machine learning is this really big tool kit and has a bunch of different things that we're going to be working with. Todd, can you tell me a little bit because you start and you teach this class, it's kind of an introductory level course on machine learning. Tell me about what you're teaching and kind of how you go about it and what are the students like about that course? What are the challenges with it?
Todd SchifelingThanks, Drew. I feel so lucky to be able to teach this course because I get to work with students from across the entire Fox School of Business. Last semester we had students from accounting, finance, marketing and supply chain management, sports tourism, hospitality management, every area of the business school. And so they come from all these different perspectives, backgrounds and interests and the questions, the research questions that they're working on.
Todd SchifelingAnd I agree with that tool kit metaphor, because that's what I try to do in the class, is provide students with lots of different tools that could be developed in lots of different ways, recombined in different ways even. And the whole point is that these are PhD students, so they're working on creating new research, new findings, new insights about the world.
Todd SchifelingAnd one of the great ways to do that is to come up with new methods. New methods enable you to answer new questions that weren't previously answerable or an answer all at the same level of insight. And so that's what students are really excited about with AML, is they can construct new variables, measure things in ways that hadn't been measured very well before and that way answer new questions.
Todd SchifelingSo this last semester, students were working on all sorts of different things related to their interests with email. They were studying public companies and their disclosures about climate change and how that matches what they're actually doing about climate change. Using text mining methods. They're studying how COVID 19 changed, how renters think about apartments and what they're interested in with apartments.
Todd SchifelingThey're studying the rise of video assistant referees, VAR Systems and soccer, and how fans react to that and understand the fairness of those systems and who's responsible for them. So, so many different methods enable students to ask and answer new questions, measure things in new ways, and create new insights, and ultimately have very successful careers from that.
Andrew ColettiBut I mean, it really seems like regardless of what field you're in, there is an application for AI and ML, and your class lets these students from all these different types of backgrounds kind of find that. Now you've told me you've taught this for three or four classes, right? So you're seeing how it's being used. Has that really made you change the way you teach it?
Andrew ColettiAs you see students’ kind of take these methods and evolve them and apply them to different areas? And if so, how have you seen that change in your class?
Todd SchifelingSo it's a really exciting time to work in this area and teach in this area because every year it becomes easier and easier. The threshold to start doing these types of analysis is declines every year as this tremendous community of researchers, developers create code and tools that are typically very open and a tremendous emphasis on collaboration and providing access, building on each other's work.
Todd SchifelingSo this is really a community in which you're encouraged to not reinvent the wheel, but take what other people have already created and run with it. And so every year, students, you know, it becomes more and more feasible for students to collect large amounts of data, construct new variables, incorporate increasingly ambitious or sophisticated techniques in their research. And I see that in the semester as well.
Todd SchifelingThat, you know, I provide some tools that we teach in the class, but then students also bring in a lot of other tools that they know about and they discover and find easy on ramps to and bring those to the class as well. So, you know, just to give you one example, like the really simple things that people are doing to understand like what the meaning of a sentence is this dictionary based approach.
Todd SchifelingYou have a list of words, and you count how many times those words were in the corpus or the paragraph or the article. And now you know something about the meaning of that text. And that's something that we still teach. Your students should understand that. But now there's all of these deep learning algorithms that are able to much more accurately detect the sentiment and meaning of sentences.
Todd SchifelingAnd those have become tremendously accessible so that students can just pull that down and incorporate that into their code and have a much more accurate sentiment, classification and analysis. Wow.
Andrew ColettiThat actually sounds really fascinating from an educators perspective because you're seeing everything that you care about that you're teaching change and be adapted and operationalized by your students in front of you. That's got to be really interesting, at least from the educator perspective. So let's talk about the student perspective. Jaeyeon, you're a student, a PhD student, and you're working a lot with AI and ML, and as Todd just said, it's changing a lot.
Andrew ColettiWhat is that like? Because I don't know if that's an experience that any other field really sees that often. So, can you talk a little bit about that?
Jaeyeon JeongI mean, as a student, I use Chester every day very often as well as I explore under tools like CloudAI and also Gemini from Google. Now I think from a research perspective, it's really allow me to explore topics more, especially for example, you find interested in the technical technicality behind the AI or the large language model. I can just ask you to explain me the out technicality of the language model, as if you're explaining to a high school student that is so easy to understand so I can personalize the information up to my needs is very efficient.
Jaeyeon JeongAnd also from a learning perspective, I generate a lot of examples to understand the concepts I really want to learn to use you very effectively for data learning. Yes, it’s a very good and useful tool, indeed.
Andrew ColettiYeah. I mean, it seems like you're making really good uses of it. And obviously, again, it's only been a few years that you're making this much use of it. So I'm sure that that's going to expand as time goes on. But you talk a little bit about research and making it easier to start your research. And you and Konstantin recently did some research on just how does the use of AI machine learning affect education?
Andrew ColettiDo you guys want to talk a little bit about that? Because I think that's an interesting concept. Yours was specifically in one field, right? It was programing, right? But obviously the ramifications go much larger. It can apply to any field. So one, you guys talk a little bit about that.
Konstantin BaumanSo on the study that we are running and what we exactly trying to understand is how the existing or large language models can affect or support students in their learning, not the quality or the task of the thing that they are producing, like the client. Right? write in the search. But you can support them, help them to write it instead of them or something.
Konstantin BaumanBut on their improved ability to do something themselves, on their improved knowledge base. So that's what we are studying. We're trying to understand like, can we find the right way toward use large language models to really support students in, in learning, not just simplifying their life to pass all the tests because things are kind of outdated. They were all developed before the existence of ChatGPT.
Konstantin BaumanNow, because we have to as educators, we have to adapt. We haven't done that so far. So we're able to eat knowledge of students based on particular tests. We need to understand like how to Egypt changes students ability to learn things. So that's what we are trying to and to understand in our study.
Andrew ColettiThat gets the same point that Todd was talking about, that it's all changing and it's changing very quickly. But we also don't necessarily have the answers yet. And I think that was kind of the result of your study, if I'm not wrong, was that the results were kind of inconclusive and that there needed to be replication and some alteration.
Andrew ColettiBut, you know, I have to be that I'm going to push a little bit further. What do you think from what you're seeing right now, where are the opportunities for it to be used better or to be how maybe assessments may get changed for the emergence of AI machine learning? How do you see education changing with this technology being used?
Konstantin BaumanIt's changing from multiple perspectives. One thing we need to do is to prepare our students for the future work. They are future employees, and as a future employee, they will use this technology here in their workplace so they have to know how to implement its let's say they need to write 100 emails during the day. It's impossible to do it right now, but with your brand, the prompt, you get the initial draft, you write each email within like half a minute and then it simplifies your work.
Konstantin BaumanIt makes you more effective. So our students need to learn how to use this technology to do a better job. Recently, I just read the paper about the copilot implemented in Github. So the test is how it affects the programmers. They run a large experiment and what the air actually doing. They're like no programmers. The time the initial line of the code and they either copilot generates the next line of the code, and they can accept, or they can change the prompt to tell what they're playing to implement.
Konstantin BaumanAnd the copilot would produce another, another code. So it's kind of helping them. And there have been very positive results from there. Like they write more effectively. The right higher quality of the code meant all the benefits are coming and.
Andrew ColettiI like what you talked about, about how it links to the career path and the field and how it's actually being curated and observed by employers. One of the things that was brought up towards the end of that workshop meeting by some of the people who work in PhD admissions was that they were seeing students who were putting on their cover letters, their resumes, that they knew how to use it, but they were actually finding they didn't totally understand it.
Andrew ColettiSo it was like they were familiar with the toolbox. But when they actually had to open up that toolbox and use it, they were picking the wrong tools. So let's talk a little bit about that. Where is the disconnect for a lot of students right now, or where are you seeing it the most? And how do we kind of adjust that so that they are coming in with the right understanding of the tools?
Konstantin BaumanSo it's a very good question. And so, it's really hard to know the exact answer for any topic. But what we see right now is that large language models, they produce some output, it's reasonable, it's meaningful. So, it can serve as a great support for a person to solve certain tasks, especially a simple task like writing a simple code which will take the least and reverse it.
Konstantin BaumanThat ChatGPT will do easily. Once you get to a more complex tasks, charge input, you can produce some output, but that would be a good first draft, so you should consider the output of charge as a support, as a first draft of what we are doing, not the final answer. So in order to be able to fix the output of ChatGPT and produce the actual answer, you need to understand what's going on.
Konstantin BaumanSo the existence of ChatGPT should not replace the need for students to know things, so they still need to learn the basic things like how to do the programing, how to write the loops. So in order to be able to check what is the output and what it's doing, yeah, it's hard to say how much they need to understand it to be able to use it, but we need to find out this balance.
Jaeyeon JeongYeah, to add on to that, because I was on the I was waiting for an admission offer like last year. So I understand why we heard that criticism comes from because as a like a candidates, I am pressured to like demonstrate that I know everything about Python. I'm ready to the statistical testing. But then I think once I got into the program, I think what I'll really program is about give engagement of how you use that method to solve a unique creative problems.
Jaeyeon JeongAnd then I think that disappointment from the maybe faculty comes from that, Oh, you know, you knew that and you told us that, you know, the python, but you haven't really engaged with the technology to solve the problem. So I think that's where all the criticism and disappointment came from this line. That is my thoughts.
Andrew ColettiYeah, and I thank you for your perspective on it. Obviously, as you just went through that, that's pretty invaluable. And Todd, I see you over there kind of like laughing a little bit. So you're the one actually teaching the course and you're around a lot of people also teaching these courses as well. Obviously, you talked about how you teach your course, but are other professors, whether at this university or at other universities or just the topic itself and how you teach machine learning?
Andrew ColettiIs it matching what we actually need to be doing for the students in actually drilling down and using it appropriately and not replacing the skills entirely? Or is it just something that's lagging a little bit behind?
Todd SchifelingYeah, I think the answers to these questions, like what we're saying depends so much on the specific context. So it's very different. Perhaps teaching undergraduate undergraduates and PhD students and the big challenges in PhD students are aligning all of the different pieces of the project that it's very easy to get so excited with the tools and we can go so far with the tools.
Todd SchifelingAnd now with ChatGPT, like really we can really push, push progress and get tremendous amounts of data constructed very quickly, for example. But ultimately to create successful and meaningful, impactful research, you have to have this really strong alignment between. We've got a great question and we've got great methods to answer that question. And so where students kind of fall off are some of the pitfalls or traps they can run into is focusing just on the tools and the methods we're putting together, you know, large data sets and constructing new variables.
Todd SchifelingBut those variables, those data don't actually answer the questions that are trying to answer or the question isn't actually interesting. So one example that I heard about from another university was, you know, this really sophisticated analysis of real estate listings and, you know, doing image recognition and processing the images and these in these ads. And ultimately the finding was that if you put pictures of plants in your real estate listing, you'll be able to have some lift in interest from buyers.
Todd SchifelingBut within that, you know, it's kind of interesting in everyday sense and certainly if you're thinking about listing a property, it's interesting to you. But from an academic perspective, from within, the discipline of knowledge that's being created, generalizable knowledge not about specific, you know, practical topics like listing your home, but a wider, more generalizable body of knowledge. That candidate wasn't successful on the job market because they weren't contributing to that larger generalizable pursuit of knowledge.
Todd SchifelingSo it's about creating the fit between the methods and the research question that that is really important at that PhD level.
Andrew ColettiAnyways, Great. Thank you. And I think you kind of got and you've given a few really good examples of how people are using it in fields that I think are a little bit less than obvious. I think when we talk about machine learning, we often kind of think and exclude it too. Coding or like data finance, right? And I think as you pointed out, there's some really cool ways that using AI machine learning, can we talk and share examples of ways that people have used it answering questions that maybe are typical for that field, but doing it in a way that utilizes new methods that are just kind of outside of the norm that people
Andrew Colettimaybe don't know about?
Konstantin BaumanThere are so many different applications of jobs and other large language models now, like the the basic thing is to get the knowledge you can take, let's say your internal documents in your company, if you did too, were large language models. And then any employee can, instead of like searching through all through the detour, they can chart with large language models and get the answers.
Konstantin BaumanAnd so.
Andrew ColettiTodd, you presented a list of different students who have used it in a really wide range of fields. I mean, to me, the one that always stood out where students in stage, that just doesn't seem like a field that's naturally is applicable to machine learning. Yet there were dozens of articles that you presented that your students had written.
Andrew ColettiI think that's fascinating. Do you have any examples you want to share?
Todd SchifelingYeah. I mean, that's again, what's really exciting about working in this area and teaching this class is getting to hear about all of the different interesting projects and directions that students are taking things basically to the larger background, to the class is so much of business activity is being digitized and digital texts or videos or images and all of that is now your data as oil.
Todd SchifelingAll of that is now possible to to analyze and extract insights from. And so if you're talking about stuff like some of the things that they're interested in are the fans around sports and how they understand what is happening in those competitions and how they organize to support teams or engage in competitions and leagues of their own. But another big area that they're interested in is the rise of sports celebrities and sports branding, both with professional athletes and now with college athletes having increasing access to those same markets to be able to to market their own brand.
Todd SchifelingSo that's another area that they're super interested in, is understanding how athletes build their brand and how fans respond to that effort, those efforts. And as well, of course, it's tourism and hospitality management. So there's a vast you can think about all the websites that are dedicated to building up and evaluating and rating tourism destinations, hospitality businesses and things like that.
Todd SchifelingSo there's a lot of research there as well. I think some of the you know, some of the things that people are pushing towards now is emphasizing video data and multimodal data. So, for example, people in finance and accounting are really interested in these conference calls where managers meet with analysts to discuss the performance of their company.
Todd SchifelingThese are publicly trade companies in the last quarter and previously people focused on text mining of the transcripts of those conference calls, but now they can also bring in the multimodal data about the facial expressions and the audio tones associated in those data and try to tease out signals about, for example, how sincere are the managers. Are they is there something they're hiding from, from analysts?
Todd SchifelingAnd can we try to explain that behavior and the consequences of that behavior? So basically, in every field, in every subject of interests, not just in management researchers, but within management and the business school, every field there's there's a lot of tremendous amount of new opportunities for developing these methods.
Andrew ColettiThat sounds like quite a range. Jen, You're obviously on the ground floor with all the other PhD students researching. Is anybody, including yourself? Any research that's standing out to you is really new and kind of doing things in a different way? Yeah.
Jaeyeon JeongI, I wanted to mention something like a Janet hour, how commerce utilized and really so for example like Amazon already adopted the journal I to summarize the reviews because there are so vast amount of reviews but also the like Shopify in the back and they help sellers to generate like a product photos and their product description with Jenny to increase the sales.
Jaeyeon JeongNow also there are some interesting research on like so using chat bot but have chapter sales salesmen personality are like outgoing sociable so if that impacts your sales as well. So I think also how I can assist in medical diagnosis and and B2B negotiation and stuff. But there are a lot of resources and real world examples are going on.
Andrew ColettiYeah, I mean, you guys presented a bunch of different examples of how people are using it in completely different but also similar ways. And I think one of the things I found interesting, you mentioned ecommerce, how that's going to be completely structured around data mining and AI machine learning. So that seems really interesting. As we kind of wrap up, I was going to ask, you know, where do you guys see AI machine learning going in education?
Andrew ColettiBut I think you've already given a pretty good perspective on that. So let's talk a little bit more just for the individual, because now obviously, as you've all pointed out, you can use it in any field. You just need to find the right question and the right methodology for it. So for those that might be interested in whatever field they're in, where might be a good place to get started learning about machine learning.
Andrew ColettiBesides obviously Constantine's wonderful workshop that he runs every single month, where else can people look to get a little bit more information on machine learning?
Todd SchifelingWell, within within Fox or Temple, they should definitely attend the workshop. And then my class is offered every fall semester, two PhD students from across Temple University. And again, like the the great thing about this community is there's so much collaboration and sharing of resources, so there are so many totally free materials available online and tutorials and so many different on ramps.
Todd SchifelingI think what I find in my class is that, you know, with education, the big challenge is motivation. How do you motivate before you can learn anything, you have to be motivated to engage. And so there is a value to a workshop or a class structure to give students help. Students build that motivation to continue to engage and build with it.
Todd SchifelingAnd then part of that, the most important thing is to find questions you're that you're interested in, that you think these methods can help you with, so that you're not just learning the methods on their own, but you're learning them towards this end of answering a question and and that's, you know, that's super important to to I think to stick with it and to make progress.
Todd SchifelingAnd the more you do, the more your horizons open, the more new questions you can envision and the more methods you become familiar with. And so it can really if you if you take that approach of like, let me find challenges that help me that that are aligned with building these skills that can really expand from there and grow over time.
Konstantin BaumanGreat. I was going to say almost the same thing. Like if you want to learn the technology you start, you need to start working with the technology and I taught that to my students in the class even before chargeback was introduced to start playing with it. And the following thoughts comment like It's very important to find some sort of a project or something which would really motivate you to do the work.
Konstantin BaumanNow, as an example, I can I had a student in the previous semester, undergraduate student. He was very interested in one game I French and I don't remember the name of the game and the story behind it with lots of characters and the interactions and so on. And he developed the he fine tuned the credibility model to be able to accurately answer the questions about the story behind each of the characters.
Konstantin BaumanAnd he's a big fan of the game and he was really into that and he like really worked in the project and developed a tool which was helpful for all the fan base.
Andrew ColettiI got to do that for all my favorite video games now too, so.
Konstantin BaumanOkay, we can discuss it in the next webcast.
Andrew ColettiHow about you?
Jaeyeon JeongJan Oh, well, I think to Professor is another dimension of our cool resources. So just so suggest interact with chat you a lot and also at Gemini because it's really fun just to like generate this photo for me. So use it as an assistant and have fun with it. And I think that could be a good starting point.
Andrew ColettiYeah, well, thank you guys all for everything you've said. Just to recap real quick, it just sounds like regardless of what field you're in, if you have a good question, there's a methodology out there using AI and ML to get the answers that you're looking for. And then it really seems like the best way to get involved is just to get involved, start working with it and as you said, maybe even work with a couple of different chat bot prompts and everything like that to really get involved.
Andrew ColettiI want to thank you all for being on this episode of the Tea and Teaching and discussing machine learning.
Konstantin BaumanYeah, thank you.